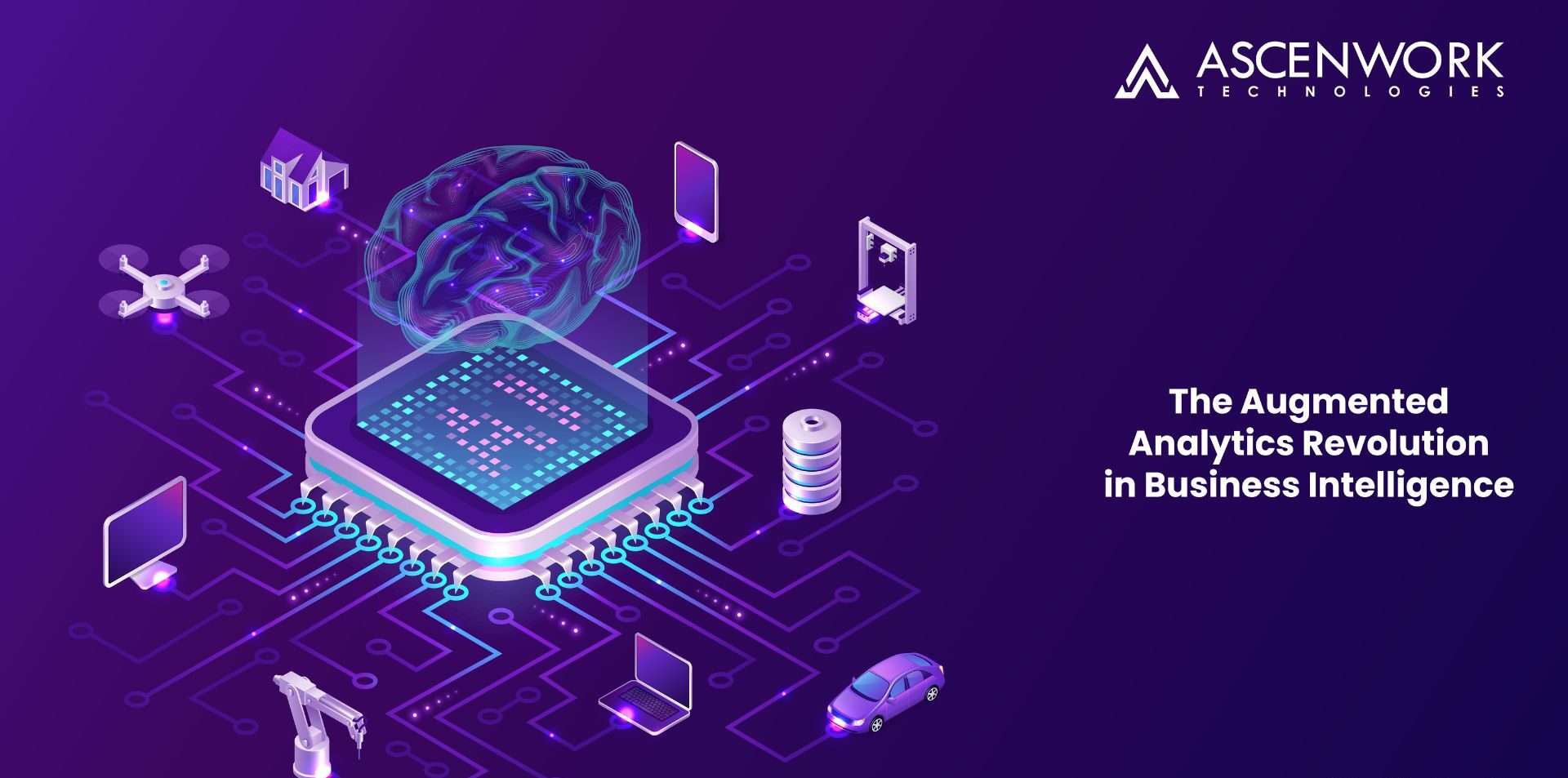
In today’s data-driven world, businesses are constantly seeking ways to extract meaningful insights AI in BI from their data. Augmented Analytics, powered by artificial intelligence, is rapidly transforming the way we approach Business Intelligence (BI). This article delves into the fascinating realm of Augmented Analytics and how it is Enhanced Insights of BI to drive better decision-making and competitive advantage.
Augmented Analytics and its core principles.
Augmented Analytics is an advanced data analytics approach that integrates artificial intelligence (AI) and machine learning (ML) technologies into the process of business intelligence (BI) and data analysis. Its core principles are centered around automating and enhancing various aspects of data analysis to make it more accessible, insightful, and valuable. The core principles of Augmented Analytics can be summarized as follows
Automation
Machine Learning and Predictive Analytics
Smart Data Discovery
Natural Language Processing
Data Visualization
Collaboration and Sharing
Benefits of Augmented Analytics in BI
Improved Accuracy
Enhanced Predictive Analytics
Democratized Data Access
Real-time Analytics
Anomaly Detection
Faster Insights
Automation of Routine Tasks
Personalization
Scalability
Cost Efficiency
AI technologies are integrated into traditional BI systems to enhance analytics
AI can automate data preprocessing tasks, such as data cleansing, imputation of missing values, and data normalization. This ensures that the data used for analysis is of high quality and accuracy.
AI algorithms can help in integrating data from various sources by identifying patterns and relationships in diverse data sets. This ensures that all relevant data is available for analysis.
AI-driven recommendation engines can suggest relevant products, content, or actions to users based on their historical behavior and preferences. This enhances user engagement and drives business growth.
AI can perform complex analytics, such as clustering, regression analysis, and segmentation, to uncover hidden Enhanced Insights within the data. This allows for a deeper understanding of customer behavior and market dynamics.
Potential challenges and the importance of data ethics and privacy
While integrating AI technologies into traditional BI systems offers numerous advantages, there are also potential challenges and concerns that organizations need to address. Additionally, data ethics and privacy are critically important considerations in the context of AI-enhanced analytics
- Data Quality and Bias: AI systems heavily rely on data for training, and if the data used is of poor quality or contains biases, the AI models can produce inaccurate or biased results. Addressing data quality and bias is crucial to ensure the reliability of AI-driven insights.
- Complex Implementation: Integrating AI into existing BI systems can be technically complex and may require specialized expertise. Organizations may face challenges related to integration, compatibility, and infrastructure upgrades.
- Data Security: AI technologies require access to sensitive and valuable data. Protecting this data from unauthorized access, breaches, or cyberattacks becomes even more critical when AI is involved.
- Regulatory Compliance: Many industries are subject to strict regulations regarding data privacy, such as GDPR in Europe or HIPAA in healthcare. Adhering to these regulations while using AI for analytics can be a challenge, as AI often involves complex data processing.
- Cost and Resource Allocation: Implementing AI technologies can be costly, and organizations need to allocate resources for training, maintenance, and ongoing monitoring of AI systems.
Importance of Data Ethics and Privacy
Trust and Reputation
Consumer Privacy
Transparency
Legal Compliance
Fairness
Security
Key Features of Augmented Analytics Tools
Augmented analytics tools represent the next generation of analytics solutions that leverage artificial intelligence (AI) and machine learning (ML) to automate data preparation, analysis, and insights generation. These tools aim to make data analytics more accessible and actionable for a broader range of users.
Automated Data Preparation
Smart Data Discovery
Automated Machine Learning
Collaboration and Sharing
Augmented Analytics is a branch of analytics that leverages artificial intelligence and machine learning to automate data preparation, insights generation, and reporting. Unlike traditional analytics, which often requires manual data handling and complex queries, Augmented Analytics makes data analysis more accessible, intuitive, and actionable.
Augmented Analytics is not limited to larger enterprises. Many Augmented Analytics tools are designed to be scalable, making them suitable for businesses of all sizes. Small businesses can benefit from these tools by gaining more accurate insights, saving time, and making data-driven decisions without the need for extensive in-house data expertise.
Augmented Analytics platforms must prioritize data privacy and security. They often adhere to data protection regulations and best practices. User permissions, data encryption, and anonymization techniques are commonly used to ensure data remains secure and compliant with privacy laws.
Augmented Analytics has a wide range of practical applications. For example, it can help sales teams identify potential leads, marketing teams refine their campaigns, finance departments forecast financial trends, and supply chain managers optimize inventory. Many industries, including healthcare, finance, and e-commerce, are using Augmented Analytics to streamline operations and improve decision-making.
While Augmented Analytics offers significant advantages, it’s not without challenges. Some limitations include the need for quality data, potential biases in AI algorithms, and the requirement for adequate training and integration. Businesses should also be aware of the ethical and regulatory considerations surrounding data use and AI-driven decision-making when implementing Augmented Analytics solutions.